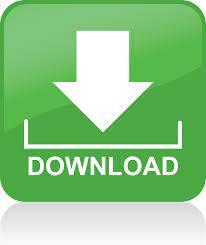
Found that just a few eigenvectors are the important ones. Predictive analytics uses a variety of statistical techniques (including data mining, machine learning, and predictive modeling) to understand future occurrences.

Carry out a principal components analysis using SAS and Minitab. Easy to use - Minitab has a highly intuitive interface designed to complement how people learn statistics.
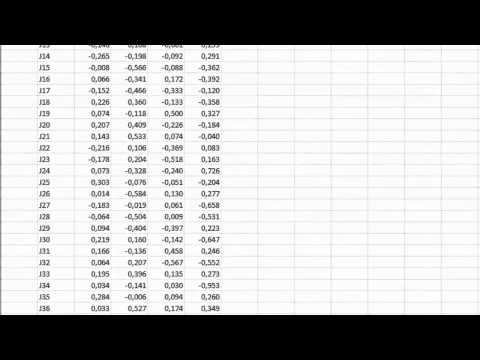
PCR is very similar to ridge regression in a certain sense. Retain the principal components that explain an acceptable level of variance. Therefore, this component focuses on an applicant's long-term financial stability.
#Multiple r squared xlstat software
PCR (Principal Components Regression) is a regression method that can be divided into three steps: The first step is to run a PCA (Principal Components Analysis) on the table of the explanatory variables, Then run an Ordinary Least Squares regression (OLS regression) also called linear regression on the selected components, The software uses a point-click interface which increases its effectiveness in statistical data analysis. At first instance, greywater collection (three times a week) for a period of three months from the informal settlement of the study area was the primary focus. The administrator wants enough components to explain 90% of the variation in the data.
#Multiple r squared xlstat how to
Many of these regression examples include the data sets so you can try it yourself! The paper uses an example to describe how to do principal component regression analysis with SPSS 10.0: including all calculating proces … The third principal component has variance 0.1474 and accounts for 3.7% of the variance in. Despite all these similarities, there is a fundamental difference between them: PCA is a linear combination of variables Factor Analysis is a measurement model of a latent variable. Principal component analysis is focused on the maximum variance amount with the fewest number of principal components. PCR is basically using PCA, and then performing Linear Regression on these new PCs. By manually setting the projection onto the principal component directions with small eigenvalues set to 0 (i.e., only keeping the large ones), dimension reduction is achieved.
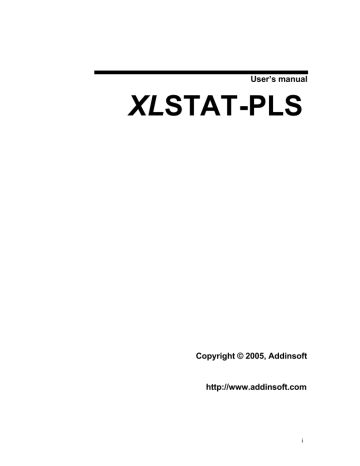
In this tutorial, we will start with the general definition, motivation, and applications of a PCA, and then use NumXL to carry on such analysis. Principal Component Analysis Consider using stepwise regression, best subsets regression, or specialized knowledge of the data set to remove these variables. Step 5: prepare data for 2nd regression model with principal components Principal Component Analysis The central idea of principal component analysis (PCA) is to reduce the dimensionality of a data set consisting of a large number of interrelated variables, while retaining as much as possible of the variation present in the data set. with population variance-covariance matrix. Brush graphs to explore points of interest. This can help identify the relationships in a dataset that are not immediately apparent. In multiple linear regression we have two matrices (blocks): X, an N × K matrix whose columns we relate to the single vector, y, an N × 1 vector, using a model of the form: y = Xb. (regression) scores with factors 0.83 0.86 0.86 0.77 # Multiple R square. Principal Component Analysis, or PCA, is a dimensionality-reduction method that is often used to reduce the dimensionality of large data sets, by transforming a large set of variables into a smaller one that still contains most of the information in the large set.
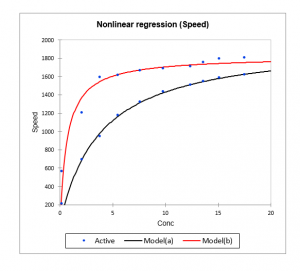
3.2 Principal Component Regression The principal components technique can be used to reduce multicollinearity in the estimation data. We can do different methods of Multivariate Analysis in Minitab such as Principal Components Analysis, Factor Analysis, Item Analysis, Cluster Analysis, Discriminant Analysis and Correspondence Analysis. Assess how many principal components are needed Interpret principal component scores and describe a subject with a high or low score Determine when a principal component analysis should be based on the variance-covariance matrix or the correlation matrix Use principal component.
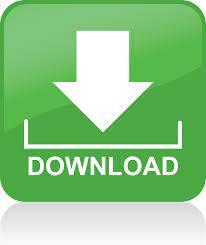